According to Google, Med-Gemini, their cutting-edge medical AI, outperforms OpenAI’s GPT-4 in factual accuracy, reliability, and handling the intricacies of clinical reasoning.
In collaboration with Google DeepMind, Google Research unveiled a new paper (published on Arxiv) detailing Med-Gemini, their upcoming AI tool designed for the healthcare field. Though currently undergoing research, Med-Gemini leverages cutting-edge technology, exceeding even established industry benchmarks, according to Google researchers.
Med-Gemini boasts large multimodal models (LMMs), all designed for different purposes and applications. Google’s Gemini models were, by default, well-armed with advanced technologies. They could process information from text, images, video, and audio. Med-Gemini is far more efficient as it’s fine-tuned with all these specialties.
Twitter / BluechipAI | Build on zkSync @Bluechip_AI
Med-Gemini comprises large multimodal models (LMMs), each tailored for specific healthcare tasks and applications. Building upon Google’s powerful Gemini (previously Bard) models, which inherently handle text, image, video, and audio data, Med-Gemini leverages this foundation with further fine-tuning for specialised medical domains. Some of these include:
Ability to Access, Process Information, And Self-Train Capabilities: Med-Gemini uses its web search capabilities to augment its advanced clinical reasoning abilities. Demonstrating its prowess, Med-Gemini achieved state-of-the-art (SOTA) performance on 10 out of 14 medical benchmarks tested, surpassing the GPT model family across all comparable metrics.
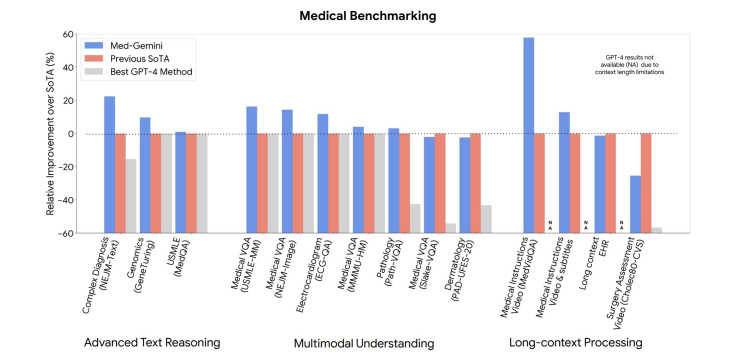
Twitter / Alex Banks @thealexbanks
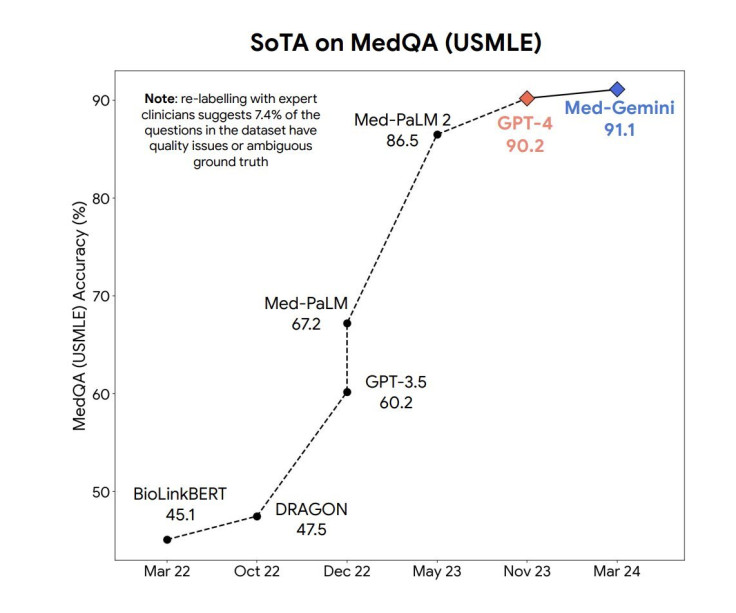
Twitter / Alex Banks @thealexbanks
According to New Atlas, Med-Gemini’s performance on the MedQA (USMLE) benchmark stands out, achieving an impressive 91.1 percent accuracy. This feat is attributed to its innovative uncertainty-guided search strategy, surpassing Google’s medical LLM, Med-PaLM 2, by a significant margin of 4.5 percent.
Streamlining Analysis of Extensive EHRs: The lengthy nature of electronic health records (EHRs), often containing repetitive text, can hinder efficient information retrieval. Researchers devised a “needle in a haystack” task to showcase Med-Gemini’s prowess in addressing this challenge.
Researchers used a massive public database called MIMIC-III (Medical Information Mart for Intensive Care). This database contains de-identified health data from intensive care unit (ICU) admissions. The task designed to test Med-Gemini’s capabilities mimicked a real-world challenge: Pinpointing a rare or subtle medical condition, symptom, or procedure buried within a vast collection of patient EHR data.
Med-Gemini performed well in the test, demonstrating its ability to extract all mentions of the target medical issue from patient records. Furthermore, it went beyond simple retrieval by assessing the relevance of each mention, categorising them, and ultimately determining a patient’s medical history with this condition. This process also highlighted Med-Gemini’s reasoning capabilities.
“Perhaps the most notable aspect of Med-Gemini is the long-context processing capabilities because they open up new performance frontiers and novel, previously infeasible application possibilities for medical AI systems,” the researchers said.
What Else To Expect?
While Med-Gemini’s capabilities are undeniably impressive, researchers acknowledge room for improvement. Nevertheless, its performance signifies a promising future for AI-powered healthcare applications.
In addition, the search giant said it will pay attention to fairness and privacy during development. “Privacy considerations, in particular, need to be rooted in existing healthcare policies and regulations governing and safeguarding patient information,” the researchers said.
Researchers acknowledge the risk of AI healthcare systems unintentionally perpetuating historical biases and inequities, recognising the importance of fairness. This isn’t just a theoretical concern; recent examples like Microsoft’s AI tool Copilot, which was criticised for generating anti-Semitic stereotypes, highlight the potential for harm.
If left unaddressed, these biases could lead to unequal model performance and potentially harmful consequences for marginalised patient groups in healthcare.